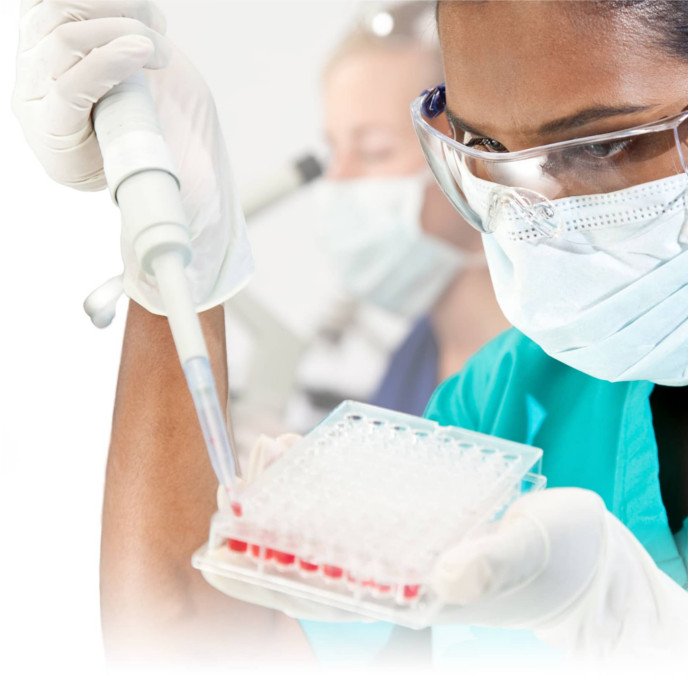
In Silico Models: Basic & Static Mechanistic Model-Based Methods for DDI Prediction
In preclinical development, a drug will be evaluated for potential to cause a drug-drug interaction (DDI) using in vitro experiments and then calculations that provide context and meaning to the results. In silico modeling using FDA-specified mathematical models provide simple and robust drug interaction risk assessment following in vitro preclinical studies.
Methods spanning simple mathematical models to complex simulations predict drug interactions based on in vitro and in vivo pharmacokinetic parameters, and in some cases replace clinical trials. Model-based methods act as a drug interaction calculator, providing a comparable metric for risk assessment. In silico DDI prediction models extrapolate in vitro data to clinically relevant enzyme- or transporter-mediated drug interactions and can also help with selection of first-in-human (FIH) dose by predicting safe drug concentrations.
There are three categories of model-based methods specified in the FDA’s Final Guidance for Industry to predict DDI risk using in vitro data:
- Simple (Basic) Static Model
- Static Mechanistic Model
- Dynamic Mechanistic Modeling (Physiologically Based Pharmacokinetic (PBPK))
Typically, an in vitro study can assess a compound’s potential to cause a DDI. Data are analyzed with appropriate in silico modeling calculations specified in the most recent FDA Drug Interaction guidance to industry to support the sponsor’s decision-making process.
Straight From the FDA Guidance: Using Model-Based Predictions to Determine a Drug’s Potential to Cause DDIs
Mathematical models can evaluate the results of in vitro and in vivo DDI studies to determine whether, when, and how to conduct further clinical DDI studies in drug development. In many cases, negative findings from early in vitro or clinical studies, in conjunction with model-based predictions, can eliminate the need for additional clinical investigations of an investigational drug’s DDI potential. Mathematical models that integrate in vitro findings and are verified with clinical PK data can play an important role in predicting the DDI potential of an investigational drug under various scenarios. There are several models to consider when evaluating the drug as a perpetrator of a metabolism-based DDI. Basic models generally serve simple purposes, such as the identification of low levels of inhibition or induction of metabolizing enzymes by an investigational drug.
Static mechanistic models can account for the disposition characteristics of both the perpetrator and the probe substrate drugs (Fahmi, Hurst, et al. 2009). Dynamic mechanistic models, including PBPK models that integrate system-dependent parameters (e.g., based on human physiology) and drug-dependent parameters (Zhao, Zhang, et al. 2011) and their time course of changes, can support decisions on when and how to conduct a clinical DDI study. Furthermore, these models can quantitatively predict the magnitude of DDI in various clinical situations, such as in patients with renal impairment or patients with genetic deficiencies in certain metabolizing enzymes.
FDA Final Guidance for Industry 2020
Basic in silico models to predict the effect of a drug as an enzyme modulator
Evaluating a drug as a potential enzyme inhibitor or inducer begins with the use of a basic model, i.e., R1, R1,gut (only for CYP3A), and R2 (only for TDI) for inhibition effect; R3, fold of change, and correlation methods for induction.
The sponsor should compare the calculated R values or fold-change to the recommended cutoff criteria to determine whether it is possible to rule out the potential for a DDI. Sponsors may calibrate their internal in vitro systems with known perpetrators and non-perpetrators of an enzyme and propose specific cutoff values with proper justification. If the basic model does not rule out the potential for a DDI, the sponsor should further evaluate the DDI potential of the investigational drug by conducting additional modeling analyses, using static mechanistic models or PBPK models or by conducting an in vivo DDI study.
FDA Final Guidance for Industry 2020
Using static mechanistic models to predict the effect of a drug as an enzyme modulator
Static mechanistic models incorporate more detailed drug disposition and drug interaction mechanisms for both interacting and substrate drugs (Fahmi, Hurst, et al. 2009). The following equation can be used to calculate the overall effect of the investigational drug on substrate drugs (represented as the AUCR).
In addition to the combination of inhibition and induction, one should separately use inhibition mechanisms to predict a drug’s enzyme inhibition potential and use induction mechanisms to predict a drug’s enzyme induction potential. It should be noted that concurrent prediction may lead to a false negative prediction if the inhibition potential is over-predicted, thus masking the induction effect. If the induction potential is over-predicted, it will mask the inhibition effect.
FDA Final Guidance for Industry 2020
Initial In Silico Modeling: Basic Model-Based Prediction Capabilities
As part of follow-up to a contracted study or as a separate consulting project, our experts can use your CYP inhibition, CYP induction, and transporter inhibition study data to evaluate DDI potential using basic or static mechanistic in silico modeling. The goal of basic models is to calculate the ratio of a victim drug’s exposure (AUC) in the presence and absence of perpetrators (inhibitors or inducers) of a drug-drug interaction.
As a follow-up to positive results from an in vitro DDI study, our team can use your compound’s dose information, Cmax (or Imax) value (the maximum plasma concentration at steady state) and fu (fraction unbound) to provide you with the following DDI risk calculations:
- R1 value for direct enzyme inhibition
- R2 value for time-dependent inhibition
- R3 value for enzyme induction
- R value for transporter inhibition ratios
Terminology for FDA / EMA / PMDA calculations
AUC = area under the curve, denotes drug exposure
AUCR = area under the plasma concentration-time curve ratio
Cmax: total maximal concentration in plasma
EC50: concentration causing half maximal effect determined in vitro
Emax: maximum induction effect determined in vitro
fu,p: unbound fraction in plasma
IC50: half-maximal inhibitory concentration
Igut: intestinal luminal concentration estimated as dose/250 mL
Iin,max: estimated maximum plasma inhibitor concentration at the inlet to the liver
Imax / Imax, u: maximal unbound plasma concentration of the interacting drug at steady state
kdeg: apparent first-order degradation rate constant of the affected enzyme
Ki: inhibition constant
KI: inhibitor concentration causing half-maximal inactivation
kinact: maximal inactivation rate constant
kobs: observed (apparent first order) inactivation rate of the affected enzyme
R: ratio of victim AUC in the presence and absence of perpetrators (inhibitors or inducers), predicted with basic models
R1: Direct (Reversible) Inhibition
R1 = 1 + (Imax,u / Ki)
R1,gut = 1 + (Igut / Ki)
Potential to inhibit if R1 ≥ 1.02 / R1,gut ≥ 11
As a follow-up to an in vitro CYP inhibition study, a sponsor may choose to further investigate direct inhibition, using the IC50 value to calculate the Ki value (or Ki can be experimentally determined), and then including unbound Imax (or Cmax) to find the R value for reversible inhibition (R1).
Further calculations by our experts can also provide insight to the critical Imax value, which denotes the threshold between a safe dose and one which could precipitate a drug-drug interaction via CYP inhibition.
R2 Time-Dependent Inhibition
R2 = (Kobs + kdeg) / kdeg
Potential to inhibit if R2 ≥ 1.25
To calculate the R value for time-dependent inhibition (R2), sponsors can choose to experimentally determine the KI, Kinact, and Kdeg values to demonstrate the effect of metabolism or time-dependent inhibition of drug-metabolizing enzymes. In these types of metabolism-dependent drug interaction, metabolites are likely the ones inhibiting the enzyme or it could be that there is a physical process such as binding to heme that could inhibit the enzyme. To determine risk of TDI, we can observe any inactivation of an enzyme by a drug candidate by using multiple drug concentrations and multiple preincubation time points.
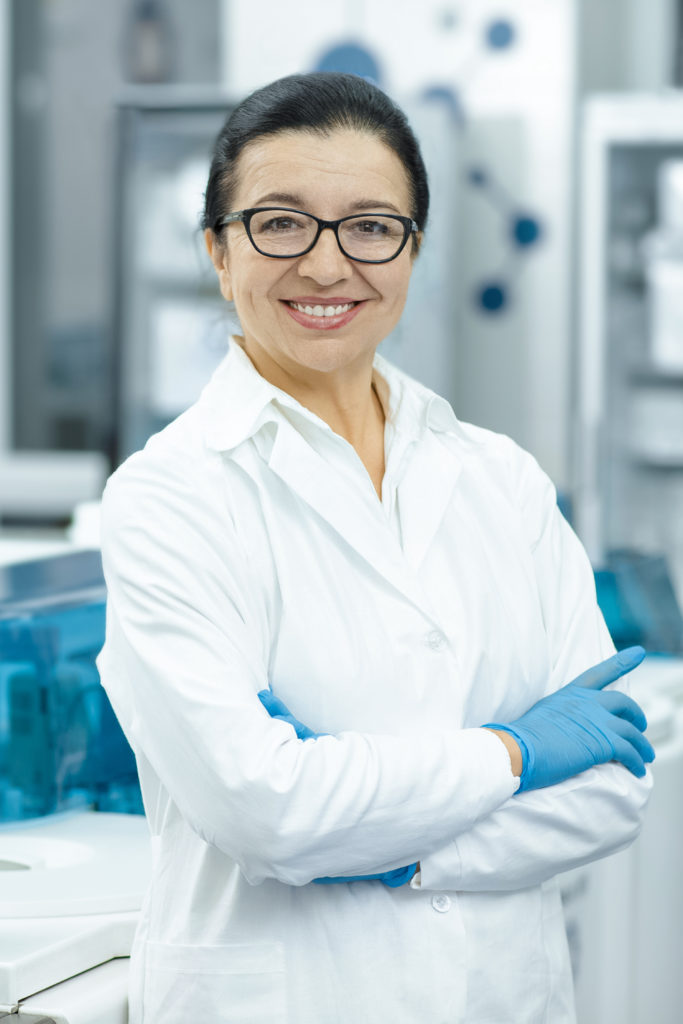
R3 Induction
R3 = 1 / [1 + (d × (Emax − 1) × 10 × Imax,u) / (EC50 + (10 × Imax,u))]
If your in vitro CYP induction study indicates a positive result in any of the hepatocyte cultures tested, the R3 can be calculated as the next step in evaluating the DDI risk and determining whether or not a clinical DDI study is necessary. Calculated R3 value is compared to a cut-off value given in the Guidance to determine drug’s induction potential in vivo.
Further calculations by our experts can also provide insight to the critical Imax / Cmax value, which denotes the threshold between a safe dose and one which could precipitate a drug-drug interaction via CYP induction. The basic model calculation involves EC50 and Emax from the in vitro study and unbound Imax / Cmax values.
In Silico prediction of transporter inhibition
To evaluate risk of transporter-mediated drug interaction, we can evaluate transporter DDI potential for each drug transporter. We can calculate the applicable ratios (R-value) that compare drug concentration in a pertinent location (e.g. intestinal lumen, hepatic inlet, etc.) to the IC50 which denotes how much drug is needed to inhibit each transporter. Different transporters have different cutoff values. Our experts can help the sponsors to ascertain the possible maximum IC50 value that will not precipitate DDI in vivo, which can impact drug dosing in the clinic.
Calculations and criteria for evaluation of inhibition of drug transporters:
Transporter | Ratio | Potential to inhibit if… |
---|---|---|
P-gp | Igut / IC50 | Ratio ≥ 10 |
BCRP | Igut / IC50 | Ratio ≥ 10 |
OATP1B1 | 1+ (fu,p × Iin,max) / IC50 | Ratio ≥ 1.1 |
OATP1B3 | 1+ (fu,p × Iin,max) / IC50 | Ratio ≥ 1.1 |
OAT1 | Imax,u / IC50 | Ratio ≥ 0.1 |
OAT3 | Imax,u / IC50 | Ratio ≥ 0.1 |
OCT2 | Imax,u / IC50 | Ratio ≥ 0.1 |
MATE1 | Imax,u / IC50 | Ratio ≥ 0.1 |
MATE2-K | Imax,u / IC50 | Ratio ≥ 0.1 |
Improve accuracy of drug interaction calculations with Plasma Protein Binding (PPB) data
Each of these calculations take into account unbound drug concentrations; in absence of plasma protein binding (PPB) study results, our specialists will assume the most conservative estimates to comply with regulatory recommendations (100% unbound). For more accurate model-based predictions, we recommend sponsors determine the drug’s binding affinity to a tenth of a percent. We conduct in vitro and in vivo (animal) PPB studies with three different method options (rapid equilibrium dialysis, ultracentrifugation, ultrafiltration) to find out your compound’s fraction unbound (fu).
Our Static Mechanistic Model-Based Prediction Capabilities
If the basic model does not rule out the potential for a DDI, the sponsor should further evaluate the DDI potential of the investigational drug by conducting additional modeling analyses, using static mechanistic models or PBPK models or by conducting an in vivo DDI study.
FDA 2020,
“In Vitro Drug Interaction Studies — Cytochrome P450 Enzyme- and Transporter-Mediated Drug Interactions Guidance for Industry“
Following comparison of the respective R values to predefined cutoff values specified by regulatory guidance, the sponsor can further determine clinical DDI potential using a static mechanistic model to determine the drug’s plasma exposure ratio, AUCR. The AUCR value can offer a more robust assessment of the drug’s risk by including more parameters.
Additionally, in cases where a drug induces and inhibits the same enzyme, DDI risk may be reduced or dismissed altogether because the diametric actions compensate each other to lower net induction or inhibition effect of the drug.
Drug Interaction Calculator: AUCR
In accordance with the FDA-recommended conservative approach to evaluating drug safety parameters, the basic static models calculate R1, R2, or R3 utilizing the maximal unbound total systemic or luminal drug concentration and the experimentally derived in vitro constants (Ki, KI, Kinact, Emax, EC50) for the prediction of clinical inhibition or induction. Further, a more robust mechanistic static model (AUCR) integrates different DDI mechanisms using in vitro constants along with the following inputs to more accurately predict the effect on victim drug metabolism when administered with the perpetrator drug:
- Maximal unbound total systemic or luminal drug concentration
- Dose
- Fraction of metabolism of a victim drug
- Hepatic blood flow
- Enteric blood flow
- Fraction of absorption
- Hepatic inlet and enterocytic drug concentrations
- Extent of binding to plasma proteins
- Blood-to-plasma concentration ratio
The FDA has provided the formula to calculate the AUCR value, however, it can get complicated. When a sponsor contracts our experts’ services as part of a follow-up to an in vitro DDI study or a separate consulting project, they can have confidence that the work will be done accurately, efficiently, and with our signature consultative approach to help you work through challenges and understand your data and what it means for your molecule moving forward. Armed with experience and scholarship of drug metabolism and pharmacokinetics of xenobiotics, our experts can act as a drug interaction calculator to give you a reliable metric of DDI risk for your compound.
After a sponsor has obtained the AUCR value, they can then compare it to critical values regulatory guidance documents have published. If the value exceeds the cutoff value, the drug developer should plan to investigate further using physiologically-based pharmacokinetics (PBPK) modeling or an in vivo (human) DDI study.
Follow the Molecule: An example of risk assessment using baic & static mechanistic in silico DDI prediction models
To paint a picture of what the decision-making process looks like for DDI risk assessment of a small molecule drug when proceeding to in silico model-based DDI prediction, let’s consider a drug developer with Compound A, which is in the final laps of preclinical development and getting ready for the IND approval process.
The drug developer has contracted with us the FDA-recommended CYP inhibition and CYP induction studies with proper study design, and the results provide the sponsor with the drug’s inhibition value of IC50 and induction values of EC50 and Emax. The drug developer then provides us with some additional parameters to calculate the R1 and R3 ratios for each CYP enzyme.
The R1 basic model yields a value of 1.12 for CYP2D6 and 1.04 for CYP3A4/5, both of which exceed the 1.02 FDA-specified cutoff. The other enzymes evaluated are below the cutoff. The R3 calculation yields that only CYP3A4 and no other evaluated enzymes indicate possible induction (FDA mandates that if the R3 value from any of the three hepatocyte cultures is ≤ 0.8, then there is potential to induce).
The sponsor then should do separate AUCR calculations for inhibition of CYP2D6 and CYP3A4 and induction of CYP3A4 and compare them to cutoff values. Additionally, we can do another calculation incorporating both induction and inhibition for CYP3A4 to find the net effect. If any of the AUCR values from a mechanistic static model exceeds cutoff values, PBPK modeling or a clinical drug-drug interaction study may be warranted.
PBPK Modeling for In Silico Analysis
If AUCR values from a mechanistic static model indicate that a drug may have potential to cause a DDI, there is one more check the drug developer can do before they must conduct a costly, time-intensive clinical DDI study. Physiologically-based pharmacokinetic (PBPK) modeling provides in silico analysis & experimentation implementing a growing reservoir of clinical drug metabolism and drug interaction data.
PBPK modeling uses results from in vitro experiments and historical data to provide the most robust assessment of DDI risk short of a human study. Since our drug metabolism, drug transporter, and plasma protein binding data are commonly used in PBPK modeling, we make sure that data reports are accurate, reliable, and in the right format for use in follow-up in silico simulation & modeling.